Getting the most from your biostatistician with Dr. Maggie Kuchibhatla
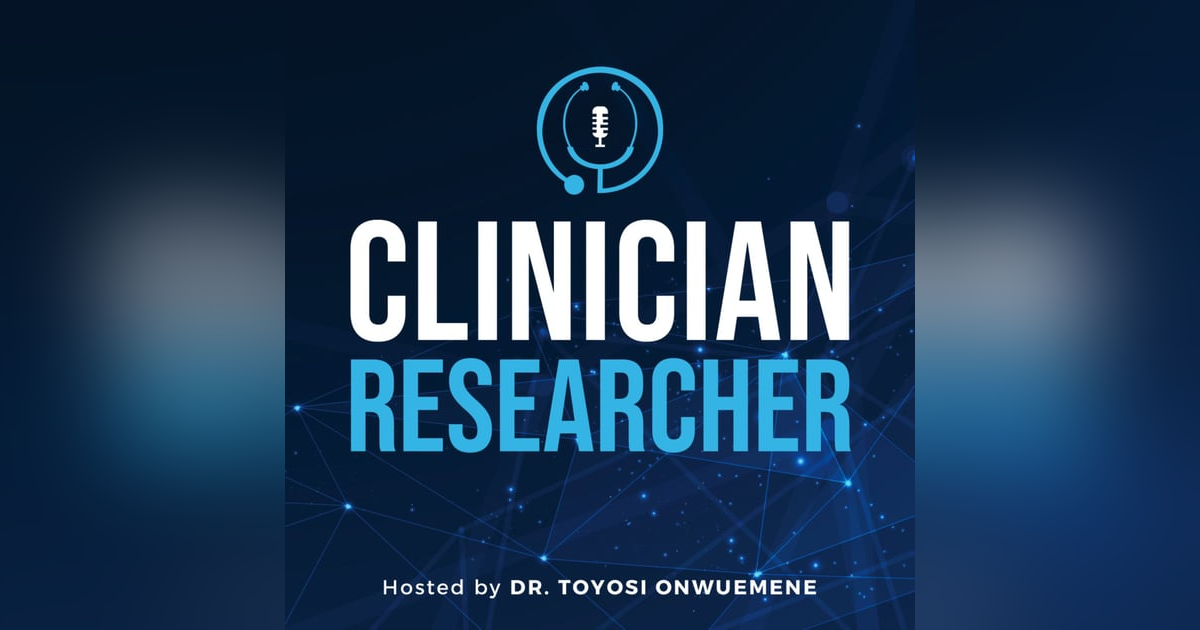
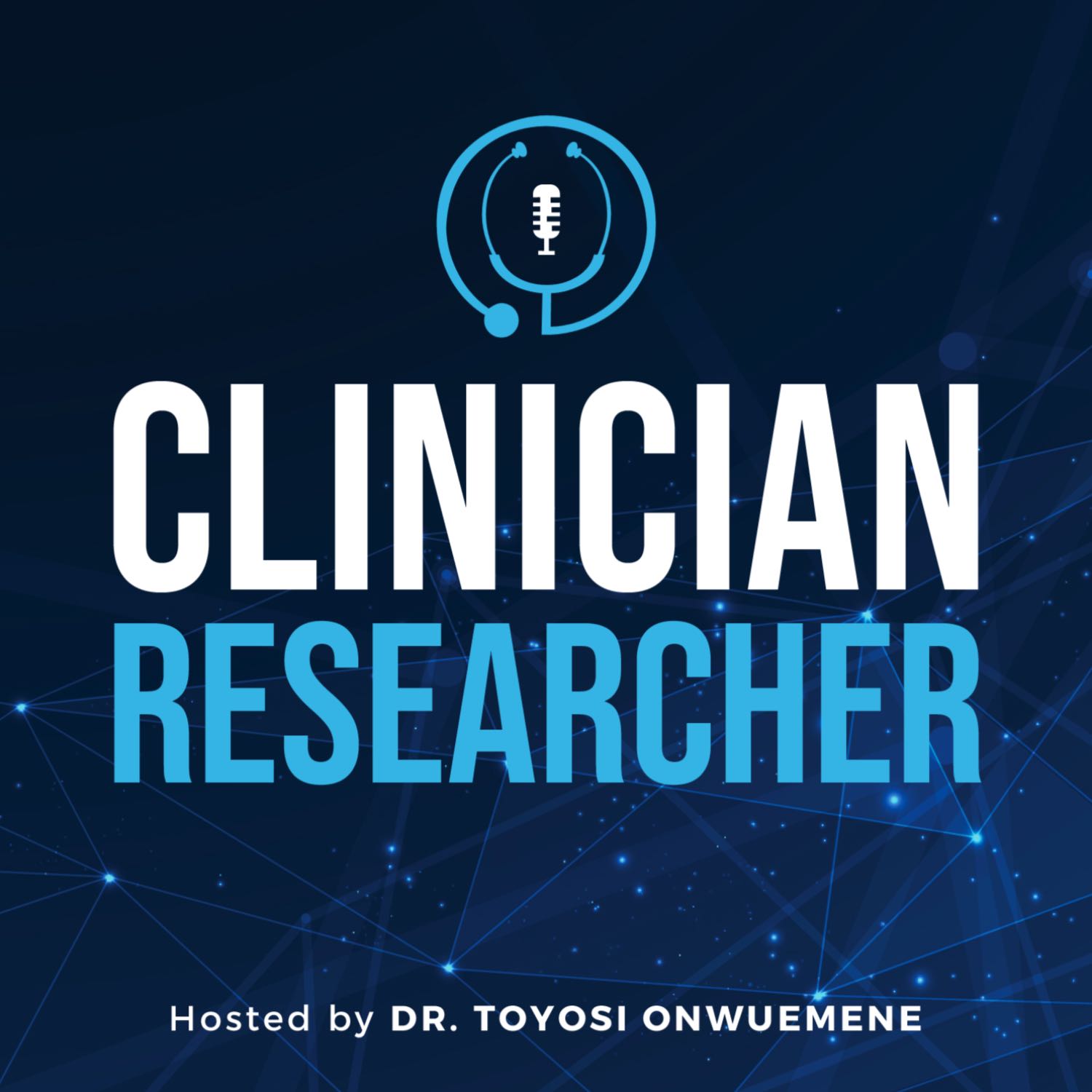
Dr. Kuchibhatla is a Professor of Biostatistics & Bioinformatics, Professor in Psychiatry and Behavioral Sciences, and a Senior Fellow in the Center for the Study of Aging and Human Development. Her expertise is in statistical research methodology, analysis of repeated measurements, latent growth curve models, latent class growth models, classification and regression trees, and designing clinical trials. Key points from our conversation are the following:
-
Engage statisticians from the outset for effective study design.
-
Statisticians enhance studies by transforming existing data into novel insights.
-
Statisticians play a vital role in crafting robust grant proposals.
-
Establish a continuous partnership with statisticians for ongoing research guidance.
Dr. Kuchibhatla underscores the collaborative synergy between statisticians and clinician researchers. Whether it's optimizing study design, innovating statistical methods, or crafting compelling grant proposals, statisticians like Dr. Kuchibhatla provide invaluable support.Are you ready to unlock the power of negotiation to amplify your research impact? If yes, sign up for Academic Negotiation Academy today: https://www.coagcoach.com/negotiation.
1
00:00:00,000 --> 00:00:05,860
Welcome to the Clinician Researcher podcast, where academic clinicians learn the skills
2
00:00:05,860 --> 00:00:11,260
to build their own research program, whether or not they have a mentor.
3
00:00:11,260 --> 00:00:17,340
As clinicians, we spend a decade or more as trainees learning to take care of patients.
4
00:00:17,340 --> 00:00:22,380
When we finally start our careers, we want to build research programs, but then we find
5
00:00:22,380 --> 00:00:27,780
that our years of clinical training did not adequately prepare us to lead our research
6
00:00:27,780 --> 00:00:29,180
program.
7
00:00:29,180 --> 00:00:35,480
Through no fault of our own, we struggle to find mentors, and when we can't, we quit.
8
00:00:35,480 --> 00:00:40,580
However, clinicians hold the keys to the greatest research breakthroughs.
9
00:00:40,580 --> 00:00:46,200
For this reason, the Clinician Researcher podcast exists to give academic clinicians
10
00:00:46,200 --> 00:00:51,800
the tools to build their own research program, whether or not they have a mentor.
11
00:00:51,800 --> 00:01:01,060
Now introducing your host, Toyosi Onwuemene.
12
00:01:01,060 --> 00:01:03,500
Welcome to the Clinician Researcher podcast.
13
00:01:03,500 --> 00:01:05,660
I'm your host, Toyosi Onwuemene.
14
00:01:05,660 --> 00:01:08,180
It is such a pleasure to be with you today.
15
00:01:08,180 --> 00:01:12,220
Thank you for listening and thank you for being here because today's an especially special
16
00:01:12,220 --> 00:01:13,220
episode.
17
00:01:13,220 --> 00:01:18,660
We have a superstar biostatistician here with us, Dr. Maggie Kuchibhatla.
18
00:01:18,660 --> 00:01:23,200
I'm going to allow her to introduce herself in a minute, but I'm so pumped because as
19
00:01:23,200 --> 00:01:27,740
clinician researchers, many times we say we have to work with biostatisticians, but we
20
00:01:27,740 --> 00:01:30,060
don't even know the first thing to do.
21
00:01:30,060 --> 00:01:34,100
And Dr. Cotubatla is going to help to kind of demystify some of that process.
22
00:01:34,100 --> 00:01:38,540
So without further ado, I'm going to invite her to introduce herself.
23
00:01:38,540 --> 00:01:41,540
Maggie, welcome to the show.
24
00:01:41,540 --> 00:01:42,540
Thank you, Tracey.
25
00:01:42,540 --> 00:01:46,380
I don't know about superstar, but I love counting numbers.
26
00:01:46,380 --> 00:01:52,340
I lived that life this year, 30 years at Duke, so, I can't complain.
27
00:01:52,340 --> 00:01:54,060
I love it.
28
00:01:54,060 --> 00:02:02,460
As a statistician, it's a pleasure to help anybody, any researcher with all kinds of
29
00:02:02,460 --> 00:02:05,820
experiences come to the door.
30
00:02:05,820 --> 00:02:15,980
But the one thing that is always helpful and will not be very upsetting for the investigators
31
00:02:15,980 --> 00:02:23,940
would be to have a basic training in research methodology.
32
00:02:23,940 --> 00:02:30,580
So there are a number of places where a young investigator can go get that kind of training.
33
00:02:30,580 --> 00:02:40,940
I mean, for example, one of the easiest places to go is the local universities offer just
34
00:02:40,940 --> 00:02:44,060
a short-term course, like a week-long course.
35
00:02:44,060 --> 00:02:50,820
And NIH offers a summer course on quantitative methods for all the investigators.
36
00:02:50,820 --> 00:02:52,460
And Johns Hopkins has a course.
37
00:02:52,460 --> 00:03:00,500
Even I, as an investigator, for some of the epidemiological stuff, I've been to Johns
38
00:03:00,500 --> 00:03:03,220
Hopkins, where they have a summer program.
39
00:03:03,220 --> 00:03:08,660
I think NIH has provided them with some funding, and they have offered those courses for anybody
40
00:03:08,660 --> 00:03:10,780
from across the country.
41
00:03:10,780 --> 00:03:13,500
And it's a small thing.
42
00:03:13,500 --> 00:03:19,780
And the institutions from where you work from can provide you a little bit of money to go
43
00:03:19,780 --> 00:03:22,540
and get that training.
44
00:03:22,540 --> 00:03:26,780
So I'll now leave it to you now to ask any questions, Toyosi.
45
00:03:26,780 --> 00:03:27,780
Sure.
46
00:03:27,780 --> 00:03:30,620
Maggie, I just want to say thank you for just starting with that.
47
00:03:30,620 --> 00:03:34,100
And something that's so important, and I know we've talked about this before, is that as
48
00:03:34,100 --> 00:03:37,100
clinicians, we get little to no research training.
49
00:03:37,100 --> 00:03:41,660
Yes, we do a little bit of research here and there, but we're not leading the projects.
50
00:03:41,660 --> 00:03:45,140
We're not deciding what's the primary outcome, what's the secondary outcome.
51
00:03:45,140 --> 00:03:46,140
And so you're right.
52
00:03:46,140 --> 00:03:49,980
When we get to our faculty positions and we're like, I now want to do research, and we're
53
00:03:49,980 --> 00:03:52,340
like, well, you've got to talk to the biostatistician.
54
00:03:52,340 --> 00:03:53,860
Sometimes we don't have a clue.
55
00:03:53,860 --> 00:03:58,260
And so I appreciate what you talk about, the importance of getting a little bit of an education,
56
00:03:58,260 --> 00:04:03,380
no matter how small it is, and research methods, so that you can have a conversation.
57
00:04:03,380 --> 00:04:07,700
In a sense, it's just, how can we even start talking when we're not speaking the same language?
58
00:04:07,700 --> 00:04:12,580
And so thank you for sharing that and how important it is for clinicians to get educated
59
00:04:12,580 --> 00:04:16,920
so that they can really contribute to the research conversation.
60
00:04:16,920 --> 00:04:21,360
So the first question I want to ask you, if you don't mind, is can you talk to me about
61
00:04:21,360 --> 00:04:25,860
what is one thing about your role that every clinician researcher should know?
62
00:04:25,860 --> 00:04:27,660
We hear about biostatisticians.
63
00:04:27,660 --> 00:04:31,980
People are always talking about biostatisticians, but what should we know about you that we
64
00:04:31,980 --> 00:04:33,460
may not know?
65
00:04:33,460 --> 00:04:41,940
Well, the one thing that the investigator should know before coming to me is know a
66
00:04:41,940 --> 00:04:48,300
little bit about what their aims are, what their goals are.
67
00:04:48,300 --> 00:04:54,980
But even before they know their aims and goals, they've got to be working on those aspects
68
00:04:54,980 --> 00:04:56,780
before coming to me.
69
00:04:56,780 --> 00:05:03,580
So in that sense, they have to know, for example, what is their study design?
70
00:05:03,580 --> 00:05:07,780
What is it that they, how are they going to answer the questions?
71
00:05:07,780 --> 00:05:13,900
Are they just going to go collect the data that is there in the literature, or in which
72
00:05:13,900 --> 00:05:17,860
case it's just a systematic study?
73
00:05:17,860 --> 00:05:24,980
Or are they going to answer a question by doing a clinical trial?
74
00:05:24,980 --> 00:05:27,720
So they need to know the study design.
75
00:05:27,720 --> 00:05:32,260
So the question is, what kind of study design are they talking about?
76
00:05:32,260 --> 00:05:39,260
Is that a clinical trial, or is it a retrospective study, or a prospective study, or an observational
77
00:05:39,260 --> 00:05:47,100
study, or is that basic science study where you're doing experiments in animals?
78
00:05:47,100 --> 00:05:55,020
Or are they doing, collecting data from running a lot of analyses from the blood?
79
00:05:55,020 --> 00:06:00,740
In other words, it's the omics, proteomics, metabolomics, genetics.
80
00:06:00,740 --> 00:06:04,820
So it's all the blood, all the data that you're getting from the blood.
81
00:06:04,820 --> 00:06:12,500
Now you can have a study where it's a combination of long-term outcomes or long-term outcomes,
82
00:06:12,500 --> 00:06:17,340
short-term outcomes, as well as all these blood work data that you're having.
83
00:06:17,340 --> 00:06:19,180
So it's a combination of all that.
84
00:06:19,180 --> 00:06:25,180
So to know, even to know what you want, you have to know what a study design is.
85
00:06:25,180 --> 00:06:28,180
So the place to go get that is, you can come to us.
86
00:06:28,180 --> 00:06:34,100
We can give you a five-minute list bill, or we can give you a lot of articles to read.
87
00:06:34,100 --> 00:06:40,700
But a good place to start would be to get a short course, because before you're coming
88
00:06:40,700 --> 00:06:45,180
to the statistician, you already know what you want, what questions to be answered.
89
00:06:45,180 --> 00:06:50,940
But how to design the study comes from going to a short course and getting to know what
90
00:06:50,940 --> 00:06:53,860
are all the study designs that are available out there.
91
00:06:53,860 --> 00:06:56,200
How do you ask a question?
92
00:06:56,200 --> 00:06:58,460
What is your outcome?
93
00:06:58,460 --> 00:06:59,700
And what else can you ask?
94
00:06:59,700 --> 00:07:02,580
What is your, what are the groups that you're comparing?
95
00:07:02,580 --> 00:07:04,980
Are you comparing just one group?
96
00:07:04,980 --> 00:07:07,260
Are you comparing several groups?
97
00:07:07,260 --> 00:07:08,700
Then what is your sample size?
98
00:07:08,700 --> 00:07:14,340
Do you have a lot of money from which you can collect the data to answer your questions?
99
00:07:14,340 --> 00:07:17,020
Or is it going to be a very small study?
100
00:07:17,020 --> 00:07:23,820
So you need to know what your sample study size is going to be and things like that.
101
00:07:23,820 --> 00:07:28,980
And then the kind of data that you're collecting, is that quantitative?
102
00:07:28,980 --> 00:07:29,980
Is that qualitative?
103
00:07:29,980 --> 00:07:30,980
Or is that ordinal?
104
00:07:30,980 --> 00:07:33,680
These are all basic stuff.
105
00:07:33,680 --> 00:07:38,540
So it doesn't need, for some statistics can be scary.
106
00:07:38,540 --> 00:07:39,540
It's not.
107
00:07:39,540 --> 00:07:44,100
It's, it can be dumbed down to one, two, three.
108
00:07:44,100 --> 00:07:45,900
Is that a quantitative, continuous one?
109
00:07:45,900 --> 00:07:46,900
Is that ordinal?
110
00:07:46,900 --> 00:07:48,620
Or is that a discrete one?
111
00:07:48,620 --> 00:07:52,900
So they're all, all these can be described to anybody.
112
00:07:52,900 --> 00:07:56,300
These days, even the 10th graders are doing statistics.
113
00:07:56,300 --> 00:07:57,300
They're doing AP statistics.
114
00:07:57,300 --> 00:08:02,180
When my daughter was going to do AP statistics, knowing her background and what she's interested
115
00:08:02,180 --> 00:08:05,420
in, I said, don't do AP statistics yet.
116
00:08:05,420 --> 00:08:08,340
Do statistics before you go to AP statistics.
117
00:08:08,340 --> 00:08:11,300
So stats are being offered at all levels.
118
00:08:11,300 --> 00:08:19,420
And I get calls from all the local schools asking, Karen, can a student just follow you
119
00:08:19,420 --> 00:08:22,660
or just to know what kind of work that you do?
120
00:08:22,660 --> 00:08:27,100
Because the person wants you to, the kid wants you to research, but they don't know where
121
00:08:27,100 --> 00:08:28,100
to start.
122
00:08:28,100 --> 00:08:32,980
So there are many ways to know, offer statistics.
123
00:08:32,980 --> 00:08:36,020
And it starts with high school, for example.
124
00:08:36,020 --> 00:08:42,940
And then if somebody is already in college and is a doctor or trained to be a doctor,
125
00:08:42,940 --> 00:08:49,100
the colleges or the universities and the medical centers offer a lot of training to do research.
126
00:08:49,100 --> 00:08:50,860
So that's the place to start.
127
00:08:50,860 --> 00:08:51,860
Awesome.
128
00:08:51,860 --> 00:08:52,860
Thank you, Maggie.
129
00:08:52,860 --> 00:08:57,140
You know, one of the things I hear you saying is that you've got to know what you want.
130
00:08:57,140 --> 00:08:59,460
Your statistician is not the one to tell you what you want.
131
00:08:59,460 --> 00:09:01,180
You got to figure it out.
132
00:09:01,180 --> 00:09:05,940
What kind of study do you, what kind of study design, how much data do you already have?
133
00:09:05,940 --> 00:09:07,500
Or what data are you collecting?
134
00:09:07,500 --> 00:09:10,100
Do you have money to collect the data prospectively?
135
00:09:10,100 --> 00:09:11,100
Is this retrospective?
136
00:09:11,100 --> 00:09:12,940
You've got to have a plan.
137
00:09:12,940 --> 00:09:15,540
And it sounds like that's where you're able to help the most.
138
00:09:15,540 --> 00:09:19,580
When somebody comes to you with a plan, they know what their primary outcome is.
139
00:09:19,580 --> 00:09:22,980
They know what they're looking for, what they're really, what questions they're seeking to
140
00:09:22,980 --> 00:09:24,740
answer from what data.
141
00:09:24,740 --> 00:09:29,260
And so it sounds like I'm hearing you say, you got to be prepared for these conversations.
142
00:09:29,260 --> 00:09:30,260
Is that fair to say?
143
00:09:30,260 --> 00:09:31,260
Yeah, it is.
144
00:09:31,260 --> 00:09:32,260
It is.
145
00:09:32,260 --> 00:09:36,140
And they turn around, they turn around to do research in a short while when they come
146
00:09:36,140 --> 00:09:39,220
with questions and they've already, they already have their groundwork done.
147
00:09:39,220 --> 00:09:45,620
We can start, though, we can start holding hands now, yes, from ground zero, but then
148
00:09:45,620 --> 00:09:50,060
it will take a longer time to study, to have, to recent goal.
149
00:09:50,060 --> 00:09:51,060
Sure.
150
00:09:51,060 --> 00:09:53,460
It's good to have some kind of training.
151
00:09:53,460 --> 00:09:58,340
And these days, you know, all the medical schools have for one year of research or six
152
00:09:58,340 --> 00:10:00,220
months of research.
153
00:10:00,220 --> 00:10:05,060
And if that's the path one wants to take, take, use that opportunity to be, to do the
154
00:10:05,060 --> 00:10:09,380
research or go to the Institute or go to a medical school where you want to just try
155
00:10:09,380 --> 00:10:10,380
that out.
156
00:10:10,380 --> 00:10:15,100
You could try that out and then get a student to say, no, this is not what you want to do.
157
00:10:15,100 --> 00:10:17,700
You want to be doing clinical, clinical work all the time.
158
00:10:17,700 --> 00:10:18,700
That's fine.
159
00:10:18,700 --> 00:10:23,140
But at least you've bettered your feed by knowing what you need to know to do research.
160
00:10:23,140 --> 00:10:24,140
Awesome.
161
00:10:24,140 --> 00:10:25,140
Thank you, Maggie.
162
00:10:25,140 --> 00:10:26,140
Now, let me ask you a question.
163
00:10:26,140 --> 00:10:28,580
And this is not a question that I thought I would ask you.
164
00:10:28,580 --> 00:10:30,220
It just comes to my mind.
165
00:10:30,220 --> 00:10:33,100
What about the people who say, well, I've had statistics one and two.
166
00:10:33,100 --> 00:10:35,300
I don't need a biostatistician.
167
00:10:35,300 --> 00:10:38,020
What do you say to those people?
168
00:10:38,020 --> 00:10:44,900
So number crunching is different from designing the study.
169
00:10:44,900 --> 00:10:54,340
And one, just let me, let me take a step further behind actually, before you come to a number
170
00:10:54,340 --> 00:10:55,340
crunching.
171
00:10:55,340 --> 00:11:00,180
So the science has to be solid.
172
00:11:00,180 --> 00:11:04,700
One that the science has to be solid, but to get data from that scientific question
173
00:11:04,700 --> 00:11:09,020
that you have, you need to know how to collect the data.
174
00:11:09,020 --> 00:11:13,420
So if you know, if you're not a statistic, statistics is number crunching.
175
00:11:13,420 --> 00:11:15,300
It cannot be just number crunching.
176
00:11:15,300 --> 00:11:18,540
It can be how to collect the data.
177
00:11:18,540 --> 00:11:23,620
You can go wrong in many places by not designing the right study.
178
00:11:23,620 --> 00:11:27,340
So involve a statistician early on.
179
00:11:27,340 --> 00:11:31,300
Engage a statistician early on.
180
00:11:31,300 --> 00:11:33,780
People like me come free.
181
00:11:33,780 --> 00:11:39,540
In some institutes, you've got to pay, but places like Duke, you have a lot of resources,
182
00:11:39,540 --> 00:11:41,020
a lot of places to go to now.
183
00:11:41,020 --> 00:11:46,660
I mean, I agree that 10 years ago, things were different, but 10 years now, there are
184
00:11:46,660 --> 00:11:47,900
a lot of places where you can go.
185
00:11:47,900 --> 00:11:52,500
There are a lot of training grants that offer you places where you can learn how to do research.
186
00:11:52,500 --> 00:11:57,140
But even there, it's good to know where to start.
187
00:11:57,140 --> 00:12:03,500
You already know some things, some basic things about how to do research.
188
00:12:03,500 --> 00:12:04,500
Awesome.
189
00:12:04,500 --> 00:12:05,500
Thank you, Maggie.
190
00:12:05,500 --> 00:12:10,380
So Maggie, what I'm hearing from you is that you don't just finish all the data collection,
191
00:12:10,380 --> 00:12:15,060
finish your study, and then go find a statistician and say, here, crunch my numbers for me.
192
00:12:15,060 --> 00:12:19,580
What you're saying is that a biostatistician is a partner in the research process.
193
00:12:19,580 --> 00:12:23,660
And from the very beginning, where you're even thinking about, how do I design the study
194
00:12:23,660 --> 00:12:29,020
to answer this question, whether it be retrospective data, prospective, how do I design the study
195
00:12:29,020 --> 00:12:30,460
to answer the question?
196
00:12:30,460 --> 00:12:34,620
I'm hearing you say a biostatistician should be a partner with you in figuring out how
197
00:12:34,620 --> 00:12:39,260
to design the study, how to collect the data, and then how to analyze the data.
198
00:12:39,260 --> 00:12:40,260
Yes.
199
00:12:40,260 --> 00:12:41,260
Analyze the data.
200
00:12:41,260 --> 00:12:47,300
And the data that you're collecting also is going to feed into your next set of research.
201
00:12:47,300 --> 00:12:52,220
So you need to have a long-term goal.
202
00:12:52,220 --> 00:12:55,860
So in that sense, have a long-term relationship with your statistician that you're working.
203
00:12:55,860 --> 00:12:56,860
They're like tools.
204
00:12:56,860 --> 00:13:00,820
They're somebody who understands your research.
205
00:13:00,820 --> 00:13:04,180
And it's a training both ways.
206
00:13:04,180 --> 00:13:06,340
I don't know everything about science.
207
00:13:06,340 --> 00:13:07,540
So I value science.
208
00:13:07,540 --> 00:13:13,260
So I'm getting all the knowledge I can from the primary investigators like you.
209
00:13:13,260 --> 00:13:18,260
And I use that knowledge to see, okay, now this is what TOEIC wants.
210
00:13:18,260 --> 00:13:21,300
This is how I need to design a study.
211
00:13:21,300 --> 00:13:24,780
And what are the best ways to collect the data?
212
00:13:24,780 --> 00:13:27,740
And what are the efficient ways to collect the data?
213
00:13:27,740 --> 00:13:32,620
And if the part of money is large, then we go a certain route.
214
00:13:32,620 --> 00:13:39,660
If the part of money is small and we have a limited time, then we just go collect immediate
215
00:13:39,660 --> 00:13:43,100
data or immediate gratification.
216
00:13:43,100 --> 00:13:47,260
So sometimes the grant is down the corner and we don't have enough time to go collect
217
00:13:47,260 --> 00:13:50,100
the data, long-term data.
218
00:13:50,100 --> 00:13:54,620
But you can just go hone in to what you've already done and answer some questions.
219
00:13:54,620 --> 00:14:00,060
A grant based on that, turn that into a proposal and turn that out.
220
00:14:00,060 --> 00:14:01,060
I love it.
221
00:14:01,060 --> 00:14:03,860
So Maggie, one of the things I'm also hearing, and you said this earlier when you talked
222
00:14:03,860 --> 00:14:05,980
about you want to do good science.
223
00:14:05,980 --> 00:14:10,640
You're not just gathering data together to just say, oh, I said something.
224
00:14:10,640 --> 00:14:12,140
You really do want to do good science.
225
00:14:12,140 --> 00:14:14,500
You want to answer a question correctly.
226
00:14:14,500 --> 00:14:16,700
And so it's important to get it right from the beginning.
227
00:14:16,700 --> 00:14:20,500
Get someone who is partnering with you so that at the end of the day, your science is
228
00:14:20,500 --> 00:14:25,720
high quality that will actually be a contribution to the field.
229
00:14:25,720 --> 00:14:30,980
So I'm hearing you talk about just in improving the quality, you're involving a statistician
230
00:14:30,980 --> 00:14:31,980
early.
231
00:14:31,980 --> 00:14:37,080
And it also helps because if you don't have enough resources to answer all the questions
232
00:14:37,080 --> 00:14:41,220
you want to, a statistician can help you focus and say, okay, well, this is the amount of
233
00:14:41,220 --> 00:14:43,500
data this can help you get.
234
00:14:43,500 --> 00:14:47,180
And this is what it will get you to the next step and the next step and the next step after
235
00:14:47,180 --> 00:14:48,180
that.
236
00:14:48,180 --> 00:14:49,660
So I'm hearing you talk about longevity too.
237
00:14:49,660 --> 00:14:52,560
This is not just about one project and you're done.
238
00:14:52,560 --> 00:14:56,580
This is really about answering a series of questions and your statistician partnering
239
00:14:56,580 --> 00:14:58,460
with you to help you do that.
240
00:14:58,460 --> 00:14:59,460
Yes.
241
00:14:59,460 --> 00:15:05,640
So when you write a grant, you also at the end of the grant, you also have to make a
242
00:15:05,640 --> 00:15:11,140
statement on what are the future uses of this grant and where are you going to go as a
243
00:15:11,140 --> 00:15:13,340
researcher at the end of this grant?
244
00:15:13,340 --> 00:15:18,060
Okay, you're giving the institution like NIH is giving us the money.
245
00:15:18,060 --> 00:15:20,460
They like the proposal point.
246
00:15:20,460 --> 00:15:24,980
But if you don't have a plan on what you want to do using the data and using the reserves
247
00:15:24,980 --> 00:15:28,620
from the money that they're funding you with, they're not going to like it.
248
00:15:28,620 --> 00:15:33,100
So you're going to come back and say, okay, once we have all this data and all these things
249
00:15:33,100 --> 00:15:39,140
answered, what are all the paths that you're going to take after you've collected and analyzed
250
00:15:39,140 --> 00:15:40,460
and published your data?
251
00:15:40,460 --> 00:15:43,600
And next set of goals, you want to have that.
252
00:15:43,600 --> 00:15:47,460
So if you don't plan the study, this plan, the study, right?
253
00:15:47,460 --> 00:15:52,540
So you, there's a chain reaction of things that you don't do right.
254
00:15:52,540 --> 00:15:53,920
Thank you for sharing that, Maggie.
255
00:15:53,920 --> 00:15:55,820
So you mentioned grants.
256
00:15:55,820 --> 00:15:57,740
And so can I ask you about that?
257
00:15:57,740 --> 00:16:03,540
In what way can a biostatistician be helpful to an investigator who's writing a grant?
258
00:16:03,540 --> 00:16:09,100
At what point do we get you involved and how do you help us in writing grants?
259
00:16:09,100 --> 00:16:13,660
So once again, I cannot emphasize the importance of science.
260
00:16:13,660 --> 00:16:16,940
So you come with the solid science, you found evidence of this.
261
00:16:16,940 --> 00:16:22,380
Now you want to design a study to answer that, to answer that in some form or in a larger
262
00:16:22,380 --> 00:16:27,420
form, or it's a, it's a conglomeration of lots of variables that are going there.
263
00:16:27,420 --> 00:16:29,840
So you want to answer all those questions.
264
00:16:29,840 --> 00:16:35,140
So now that means again, back to, back to the drawing board, you're going to be needing
265
00:16:35,140 --> 00:16:36,820
to design the study.
266
00:16:36,820 --> 00:16:42,460
So my question would be, when, when do you want to start the study?
267
00:16:42,460 --> 00:16:45,740
So if they say that they want to start the study tomorrow, my question is, what is it
268
00:16:45,740 --> 00:16:47,460
that you have now?
269
00:16:47,460 --> 00:16:52,340
So if they already have the data now and they want to write a grant, my question would be,
270
00:16:52,340 --> 00:16:58,340
well, once you have some data and you already know that some of your questions are answered,
271
00:16:58,340 --> 00:17:05,600
but you want some more to be answered, involve the statistician early on in when you have
272
00:17:05,600 --> 00:17:11,540
your aims, your goals and your future questions to be answered.
273
00:17:11,540 --> 00:17:15,860
When you have all those things written up and you've formed it up, formed it up to some
274
00:17:15,860 --> 00:17:23,740
extent, come see a statistician because then the, the principal investigators and the statisticians
275
00:17:23,740 --> 00:17:30,440
can sit together, put their minds together and design, come up with a design that's best
276
00:17:30,440 --> 00:17:36,820
for what your aims are and what data you have and what data can be collected for the current
277
00:17:36,820 --> 00:17:39,140
study that you have in mind.
278
00:17:39,140 --> 00:17:40,140
Awesome.
279
00:17:40,140 --> 00:17:43,420
And I keep hearing you talk about the importance of that partnership.
280
00:17:43,420 --> 00:17:47,960
So have a plan, come up with your specific aims, and then let's sit together and design
281
00:17:47,960 --> 00:17:51,700
what that study will look like to fit the goals of your study.
282
00:17:51,700 --> 00:17:52,700
Yeah.
283
00:17:52,700 --> 00:17:53,700
Okay.
284
00:17:53,700 --> 00:17:56,180
So now, Maggie, let me ask you this.
285
00:17:56,180 --> 00:18:00,180
You know, you've kind of answered the question about how can clinicians come prepared to
286
00:18:00,180 --> 00:18:01,500
get value from you?
287
00:18:01,500 --> 00:18:05,900
You've talked about get a little bit of an education so we can have a conversation and
288
00:18:05,900 --> 00:18:08,460
then have a clear goal for where you want to go.
289
00:18:08,460 --> 00:18:12,500
I just wonder, is there anything else you want to add to what, how can clinicians best
290
00:18:12,500 --> 00:18:16,100
get value from the experience of working with a biostatistician?
291
00:18:16,100 --> 00:18:21,660
So, so if they're, if they're, if they're from day one, let's say day one, they have
292
00:18:21,660 --> 00:18:26,300
nothing but they have an idea and they want to do something, come to us because we can
293
00:18:26,300 --> 00:18:31,980
design the study at that time and tell you what, what to do several things.
294
00:18:31,980 --> 00:18:37,020
One, to go, we will tell the investigators to go and look into the literature and come
295
00:18:37,020 --> 00:18:39,500
up with it for the questions that they want.
296
00:18:39,500 --> 00:18:42,300
Is there anything in the literature that they have already done?
297
00:18:42,300 --> 00:18:47,220
That are there researchers who've already done that kind of research?
298
00:18:47,220 --> 00:18:50,140
If they have, what are all the results they have?
299
00:18:50,140 --> 00:18:52,980
So that, you know, get an Excel spreadsheet.
300
00:18:52,980 --> 00:18:55,660
I will tell the investigator to get an Excel spreadsheet.
301
00:18:55,660 --> 00:18:56,660
This is the question.
302
00:18:56,660 --> 00:18:59,860
These are all the, these are all the papers that are out there.
303
00:18:59,860 --> 00:19:04,660
In each of these papers, what is it that the, that that particular investigator has looked
304
00:19:04,660 --> 00:19:10,780
at and what is it that I'm going to contribute that they have not contributed to?
305
00:19:10,780 --> 00:19:14,860
Because nobody's going to give us money if something is already looked at several times
306
00:19:14,860 --> 00:19:18,580
and the results and the same results are coming up over and over again.
307
00:19:18,580 --> 00:19:23,860
So you have to, you have to come up with something that is kind of novel, over and above that
308
00:19:23,860 --> 00:19:26,420
is already studied in the literature.
309
00:19:26,420 --> 00:19:33,220
So if, if a part of that particular question that you have in mind, most of it is answered,
310
00:19:33,220 --> 00:19:38,540
but some of it is not answered, then we can help you design a study in addition to what
311
00:19:38,540 --> 00:19:47,900
is already there, how you can either add to what is already there by adding a new design
312
00:19:47,900 --> 00:19:53,020
and going and collecting data for that particular aspect of that aim that you have.
313
00:19:53,020 --> 00:19:57,460
And then move from there to the next phase.
314
00:19:57,460 --> 00:20:01,820
So what I also hear you talking about, Maggie, is that you bring the innovation too.
315
00:20:01,820 --> 00:20:06,700
So you can help people think about how to take what's already present and make it new.
316
00:20:06,700 --> 00:20:10,660
And so in, you know, for clinicians who are trying to write an innovation section of their
317
00:20:10,660 --> 00:20:13,740
grant, you actually can help with that and helping them innovate.
318
00:20:13,740 --> 00:20:16,620
Yes, because innovation is science.
319
00:20:16,620 --> 00:20:18,580
Innovation is also a new statistical methods.
320
00:20:18,580 --> 00:20:25,860
So some of the questions could not be answered, you know, in a very sophisticated way earlier
321
00:20:25,860 --> 00:20:28,380
on from 20 years ago.
322
00:20:28,380 --> 00:20:36,780
But now with the advent of computing power, immense computing powers, especially since
323
00:20:36,780 --> 00:20:42,340
the last 10 years, the immense computing power, the computing, it's not too expensive to run
324
00:20:42,340 --> 00:20:46,660
big numbers, to run big, big models.
325
00:20:46,660 --> 00:20:50,540
Ten years ago, it would take two nights to run a study.
326
00:20:50,540 --> 00:20:56,300
Or we used to, we have to go to a supercomputing center to run some models.
327
00:20:56,300 --> 00:21:02,260
But now with the advent of computers and the cheapness and how cheap they are to run some
328
00:21:02,260 --> 00:21:10,540
of these models, in terms of time, we can advocate newer methodologies that will incorporate
329
00:21:10,540 --> 00:21:15,420
lots and lots of variables from different models to come up with a very complicated
330
00:21:15,420 --> 00:21:16,420
model.
331
00:21:16,420 --> 00:21:17,420
I like it.
332
00:21:17,420 --> 00:21:18,420
Thank you.
333
00:21:18,420 --> 00:21:20,700
Innovation in the statistical methods, which is great.
334
00:21:20,700 --> 00:21:23,540
It's not something I think about, but that's absolutely necessary.
335
00:21:23,540 --> 00:21:24,540
All right.
336
00:21:24,540 --> 00:21:25,540
That's awesome.
337
00:21:25,540 --> 00:21:26,540
Okay.
338
00:21:26,540 --> 00:21:27,540
Let me ask you this.
339
00:21:27,540 --> 00:21:31,540
How, what, what is one life hack that you can share that maybe clinicians don't know
340
00:21:31,540 --> 00:21:35,660
about as what is one life hack that you have to share?
341
00:21:35,660 --> 00:21:43,700
Well, our hearts are very important to us and heart, you know, heart stops, feet all
342
00:21:43,700 --> 00:21:44,700
gone.
343
00:21:44,700 --> 00:21:50,340
So a few years ago, one of the investigators here came to me and said, Hey, we did this
344
00:21:50,340 --> 00:21:59,360
research in our, in our lab and they found this one more car to be very high in these
345
00:21:59,360 --> 00:22:03,860
patients who are going to, whose heart is going to fail.
346
00:22:03,860 --> 00:22:06,220
How do we design a study?
347
00:22:06,220 --> 00:22:09,580
How do we, how, what do we do next?
348
00:22:09,580 --> 00:22:13,540
So we, what we, we want to, we want to say that we have this four hearts out of these
349
00:22:13,540 --> 00:22:21,060
four cars, three hearts had this one marker very high, but this is not enough to get money.
350
00:22:21,060 --> 00:22:23,020
And we will start a design study.
351
00:22:23,020 --> 00:22:27,820
We just put the heart in the solution and we try to see what came out of the, from these
352
00:22:27,820 --> 00:22:30,740
hearts and we tested the solution that was there.
353
00:22:30,740 --> 00:22:36,620
The heart was put in a solution when we looked at the solution and we found this marker in
354
00:22:36,620 --> 00:22:38,540
all three of these cards.
355
00:22:38,540 --> 00:22:42,380
So I said, how, you know, my studies are cheap.
356
00:22:42,380 --> 00:22:45,380
You know, we cannot kill people.
357
00:22:45,380 --> 00:22:47,980
We only can get the hearts from people who die.
358
00:22:47,980 --> 00:22:58,300
So why don't we take the hearts of mice or if you have more money or pigs or rabbits,
359
00:22:58,300 --> 00:22:59,820
any of these.
360
00:22:59,820 --> 00:23:07,720
And we design a study where we put a stressor in the hearts.
361
00:23:07,720 --> 00:23:14,180
So they're going to die or they're going to be near that near death and see what comes
362
00:23:14,180 --> 00:23:21,860
out of their hearts because our animal models eventually do translate into human models.
363
00:23:21,860 --> 00:23:22,860
Right.
364
00:23:22,860 --> 00:23:26,340
It was some for some they are not, but for most part they're, they're pretty, pretty
365
00:23:26,340 --> 00:23:27,340
close.
366
00:23:27,340 --> 00:23:28,340
We are all pretty close.
367
00:23:28,340 --> 00:23:30,380
So why don't you, why don't we do that in the next?
368
00:23:30,380 --> 00:23:32,580
I said, how long will it take?
369
00:23:32,580 --> 00:23:34,860
The grant is due.
370
00:23:34,860 --> 00:23:35,860
This is Brookhaven.
371
00:23:35,860 --> 00:23:41,020
Brookhaven Institute, they were the, the grant is due in like six months.
372
00:23:41,020 --> 00:23:42,020
Can you do a trial?
373
00:23:42,020 --> 00:23:43,820
Can you do studies in the next two to three months?
374
00:23:43,820 --> 00:23:47,100
He said in three months we can get the result that you want.
375
00:23:47,100 --> 00:23:55,620
So they came up, we came up with some, some estimates using mice as an example.
376
00:23:55,620 --> 00:23:59,900
And it translated what we saw in the human, human anecdotal data.
377
00:23:59,900 --> 00:24:01,500
These are anecdotal data.
378
00:24:01,500 --> 00:24:02,500
We use that.
379
00:24:02,500 --> 00:24:05,780
And then we said, okay, mice models are cheaper to run.
380
00:24:05,780 --> 00:24:10,500
So let's see if we can, if we do the same thing in mice, what happens?
381
00:24:10,500 --> 00:24:14,580
So we initially we did three mice and we found some good results.
382
00:24:14,580 --> 00:24:20,260
We then moved on to a slightly larger samples, six mice, and we, the results were consistent
383
00:24:20,260 --> 00:24:23,940
with what we saw in the human hearts.
384
00:24:23,940 --> 00:24:31,820
And we had two cycles of grants that got funded based on those small studies that we designed
385
00:24:31,820 --> 00:24:37,140
in animals and then we went on to get some more grants.
386
00:24:37,140 --> 00:24:38,140
That's really awesome.
387
00:24:38,140 --> 00:24:41,900
So Maggie, I hear you, you keep reiterating the importance of getting your partners by
388
00:24:41,900 --> 00:24:46,140
statisticians involved early because they can help you think about how do you set up
389
00:24:46,140 --> 00:24:50,940
to be ready to submit a grant, to be ready to be successful in grant funding.
390
00:24:50,940 --> 00:24:54,260
But I'm also hearing you, hearing you talk about how much time is needed.
391
00:24:54,260 --> 00:24:59,380
So you were talking about investigators coming to you six months before a grant is due.
392
00:24:59,380 --> 00:25:04,260
Talk about how much time is needed to really prepare a good submission with the help of
393
00:25:04,260 --> 00:25:06,740
the statistician.
394
00:25:06,740 --> 00:25:09,420
So basic science is a different beast.
395
00:25:09,420 --> 00:25:15,540
So sure, this study we were able to do very quickly and there were a lot of resources.
396
00:25:15,540 --> 00:25:20,180
There was a big name, so the investigators have a lot of money and a lot of resources,
397
00:25:20,180 --> 00:25:25,940
so we were able to turn around and do the work and get the data to have to submit to
398
00:25:25,940 --> 00:25:26,940
the grant.
399
00:25:26,940 --> 00:25:29,060
But it's not always the case.
400
00:25:29,060 --> 00:25:33,740
The mice studies, all the mice can die for various reasons.
401
00:25:33,740 --> 00:25:36,140
And so we may not have solid data.
402
00:25:36,140 --> 00:25:38,900
So I would say, come even a year ahead.
403
00:25:38,900 --> 00:25:45,780
If you have some data, then just anecdotally, let's say you found some data anecdotally,
404
00:25:45,780 --> 00:25:50,940
then come to us right away and see how we can set up a study that we can systematically
405
00:25:50,940 --> 00:25:54,620
collect the data and write a grant on that.
406
00:25:54,620 --> 00:25:59,300
Because it's not just one question that you're going to answer from designing the study.
407
00:25:59,300 --> 00:26:01,900
You're going to be answering three or four questions.
408
00:26:01,900 --> 00:26:04,140
The aims typically are...
409
00:26:04,140 --> 00:26:11,820
So an R21 mechanism kind of helps you get data, collect data to write a big old grant.
410
00:26:11,820 --> 00:26:12,820
So it's basically R21.
411
00:26:12,820 --> 00:26:21,220
R03 is basically an R-age mechanism to really collect pilot data and then use that data
412
00:26:21,220 --> 00:26:25,780
then to do kind of an R21 and then move on to a bigger one.
413
00:26:25,780 --> 00:26:33,900
But in the medical schools, there are all these training grants that give you pilot
414
00:26:33,900 --> 00:26:35,520
money.
415
00:26:35,520 --> 00:26:41,060
So some of these small grants, $10,000 grants, can help you set up small studies.
416
00:26:41,060 --> 00:26:48,420
They may not fund your salary, but they can fund small studies, lab studies.
417
00:26:48,420 --> 00:26:49,420
Those are one.
418
00:26:49,420 --> 00:26:50,420
That is lab studies.
419
00:26:50,420 --> 00:26:57,580
But if they're not lab studies, if they involve secondary data, secondary data is already
420
00:26:57,580 --> 00:26:59,220
there.
421
00:26:59,220 --> 00:27:05,500
So this money can be the small study funding from pilot studies internally can be used
422
00:27:05,500 --> 00:27:21,940
on writing up grants, small grants, and also come up with some other decent studies that
423
00:27:21,940 --> 00:27:23,380
can go with that.
424
00:27:23,380 --> 00:27:26,580
So it doesn't have to ask just one question.
425
00:27:26,580 --> 00:27:30,300
You'll have three or four questions that can go with as part of the project.
426
00:27:30,300 --> 00:27:31,460
I love it.
427
00:27:31,460 --> 00:27:36,020
So I hear you talking about how you can even help people really maximize the benefit of
428
00:27:36,020 --> 00:27:37,380
any sample of data.
429
00:27:37,380 --> 00:27:39,780
So it's like here you're collecting this data.
430
00:27:39,780 --> 00:27:40,900
This is what you can get from it.
431
00:27:40,900 --> 00:27:41,900
You can also get this.
432
00:27:41,900 --> 00:27:43,500
You can also get that.
433
00:27:43,500 --> 00:27:48,420
And I also hearing you talk about for everyone to realize that no matter how small the pot
434
00:27:48,420 --> 00:27:52,500
of money you get, you can always do something with it to turn it into the next grant and
435
00:27:52,500 --> 00:27:54,980
the next grant and the next grant after that.
436
00:27:54,980 --> 00:27:55,980
That's awesome.
437
00:27:55,980 --> 00:27:56,980
Awesome.
438
00:27:56,980 --> 00:27:57,980
All right.
439
00:27:57,980 --> 00:28:03,020
Even big data sets, even big data sets, we could do some small studies using the big
440
00:28:03,020 --> 00:28:09,460
national databases, using the internal funding and use that to write bigger grants.
441
00:28:09,460 --> 00:28:10,460
I love it.
442
00:28:10,460 --> 00:28:11,460
I love it.
443
00:28:11,460 --> 00:28:12,460
Thank you.
444
00:28:12,460 --> 00:28:13,460
Thank you.
445
00:28:13,460 --> 00:28:14,460
Okay.
446
00:28:14,460 --> 00:28:17,980
So Maggie, if there is a clinician sitting out there thinking, I want to become a researcher,
447
00:28:17,980 --> 00:28:23,140
I want to work with a biostatistician, but I'm not sure I can, what encouragement do
448
00:28:23,140 --> 00:28:26,540
you have for them in terms of how best to move forward?
449
00:28:26,540 --> 00:28:28,260
Yeah.
450
00:28:28,260 --> 00:28:42,780
So nationally, NIH has started providing mechanisms for quantitative, to provide quantitative
451
00:28:42,780 --> 00:28:44,860
help to the institutes.
452
00:28:44,860 --> 00:28:53,820
So the CTSI is one of, one such grant that many organizations or many academic institutions
453
00:28:53,820 --> 00:29:08,540
write to get money, so that CTSI, it's like a, it's a core that helps investigators within
454
00:29:08,540 --> 00:29:14,100
the institute with all kinds of help they need.
455
00:29:14,100 --> 00:29:23,980
So Duke got two rounds of CTSI grants and as part of that, some of that money is given
456
00:29:23,980 --> 00:29:26,260
to the statisticians.
457
00:29:26,260 --> 00:29:32,180
So a 20 or 25% of a statistician's salary is covered by the CTSI.
458
00:29:32,180 --> 00:29:40,700
So those statisticians, what they do is now help investigators who are starting from ground
459
00:29:40,700 --> 00:29:42,860
zero.
460
00:29:42,860 --> 00:29:48,180
And they can tap into those aspects of any institute that they have.
461
00:29:48,180 --> 00:29:51,540
So practically every institute has some kind of money.
462
00:29:51,540 --> 00:29:55,020
It's the question of how much money that you want or how much effort that you want from
463
00:29:55,020 --> 00:29:56,660
a statistician.
464
00:29:56,660 --> 00:30:01,260
So if an investigator, if a new investigator is getting through the door, we have that
465
00:30:01,260 --> 00:30:02,260
all the time.
466
00:30:02,260 --> 00:30:08,740
We have lots of, lots and lots of investigators coming into our institute.
467
00:30:08,740 --> 00:30:14,380
And the first thing they want to know is what are the research resources available?
468
00:30:14,380 --> 00:30:20,700
So call up, so the thing to do is to call the Biostat department and find out what are
469
00:30:20,700 --> 00:30:22,700
all the resources available.
470
00:30:22,700 --> 00:30:28,620
Or within an institute, for example, Division of Hematology, for example, within the Division
471
00:30:28,620 --> 00:30:34,300
of Hematology, find out who are the, what are all the resources available to do research.
472
00:30:34,300 --> 00:30:43,220
Both basic science, long-term outcomes, outcomes research, what are all the resources available.
473
00:30:43,220 --> 00:30:44,420
So that's the starting point.
474
00:30:44,420 --> 00:30:50,740
If within the department or within the department that you're in, what are the resources available?
475
00:30:50,740 --> 00:30:52,460
And there are multiple resources available.
476
00:30:52,460 --> 00:30:54,700
That's not the only resource available.
477
00:30:54,700 --> 00:31:01,100
If the department tells you that this resource is available only for people who have funding,
478
00:31:01,100 --> 00:31:07,780
then at the School of Medicine level, there are resources available that are available
479
00:31:07,780 --> 00:31:11,300
for any researcher who can start from ground down.
480
00:31:11,300 --> 00:31:12,300
That's awesome.
481
00:31:12,300 --> 00:31:13,300
Thank you, Maggie.
482
00:31:13,300 --> 00:31:17,920
What I'm hearing from you is just that you got to keep pushing for what you need.
483
00:31:17,920 --> 00:31:21,780
Make sure you're looking, especially as you're applying for your first faculty job, making
484
00:31:21,780 --> 00:31:25,760
sure that these resources are already available, how you're going to get access to them.
485
00:31:25,760 --> 00:31:30,140
But even if you come and you don't have access to the resource to get a biostatistician to
486
00:31:30,140 --> 00:31:35,420
work with, look to your department, look to the schools, look to the institutes, especially
487
00:31:35,420 --> 00:31:39,780
if you have a CTSI, and look to see what resources are available.
488
00:31:39,780 --> 00:31:43,700
And I love that because I think one of the things, Maggie, we tell our audience is that
489
00:31:43,700 --> 00:31:45,340
you can't, don't get stuck.
490
00:31:45,340 --> 00:31:49,820
Don't make sure that you're taking ownership and leading your own research, not letting
491
00:31:49,820 --> 00:31:51,100
obstacles stop you.
492
00:31:51,100 --> 00:31:55,100
And so it sounds like really it's that there are resources available, but you do need to
493
00:31:55,100 --> 00:31:56,700
go out and look for them.
494
00:31:56,700 --> 00:31:57,700
Yeah, yeah.
495
00:31:57,700 --> 00:31:58,700
That's right.
496
00:31:58,700 --> 00:32:00,700
I do want to mention one thing though.
497
00:32:00,700 --> 00:32:07,620
So every department, if it's a research institution, every department has a vice chair of research.
498
00:32:07,620 --> 00:32:10,980
Go talk to the vice chair of research and tell them you are interested.
499
00:32:10,980 --> 00:32:16,940
You did some research in college or in high school, college, and in mid school that you
500
00:32:16,940 --> 00:32:18,500
want to pursue some of that research.
501
00:32:18,500 --> 00:32:20,940
How do I go about doing that?
502
00:32:20,940 --> 00:32:25,780
Talk to the vice chair of research and the vice chair of research will be able to help
503
00:32:25,780 --> 00:32:30,500
you put you in touch with somebody who's already doing something along those lines.
504
00:32:30,500 --> 00:32:33,540
So you have a mentoring right there.
505
00:32:33,540 --> 00:32:38,220
And so you get to start working with that particular research with somebody who's already
506
00:32:38,220 --> 00:32:39,540
doing that kind of work.
507
00:32:39,540 --> 00:32:45,620
Or you can start your own search because the department is interested in something that
508
00:32:45,620 --> 00:32:48,420
you just put up with.
509
00:32:48,420 --> 00:32:49,420
I love it.
510
00:32:49,420 --> 00:32:50,420
Thank you, Maggie.
511
00:32:50,420 --> 00:32:53,540
I hear you saying, you know what, there's someone at your institution who cares that
512
00:32:53,540 --> 00:32:54,980
research gets done.
513
00:32:54,980 --> 00:32:58,940
So find them and have them help you because that is their job.
514
00:32:58,940 --> 00:32:59,940
That is so awesome.
515
00:32:59,940 --> 00:33:03,260
Maggie, you have shared such amazing insights.
516
00:33:03,260 --> 00:33:04,260
Thank you so much.
517
00:33:04,260 --> 00:33:09,980
It is rare that we really have access to biostatisticians to help us get the inside story on how to
518
00:33:09,980 --> 00:33:11,980
work well with the biostatistician.
519
00:33:11,980 --> 00:33:15,020
And so I really want to thank you for the insights you've shared today.
520
00:33:15,020 --> 00:33:19,540
And to our audience members, if you have benefited from the things that Maggie has shared, please
521
00:33:19,540 --> 00:33:22,060
share this episode with somebody else who needs to hear it.
522
00:33:22,060 --> 00:33:26,500
Or if you're a mentor and your mentees need to understand this, please share this episode
523
00:33:26,500 --> 00:33:27,500
with them.
524
00:33:27,500 --> 00:33:31,700
So having said that, I want to say Maggie, thank you so much for coming on the show and
525
00:33:31,700 --> 00:33:34,660
sharing your wisdom with our audience.
526
00:33:34,660 --> 00:33:35,660
Thank you, Tiosi.
527
00:33:35,660 --> 00:33:37,780
Thank you for giving me this opportunity.
528
00:33:37,780 --> 00:33:41,260
And so thank you to our audience and we'll see you again next time.
529
00:33:41,260 --> 00:33:44,260
Take care.
530
00:33:44,260 --> 00:33:50,700
Bye.
531
00:33:50,700 --> 00:33:56,060
Thanks for listening to this episode of the Clinician Researcher Podcast, where academic
532
00:33:56,060 --> 00:34:01,500
clinicians learn the skills to build their own research program, whether or not they
533
00:34:01,500 --> 00:34:02,860
have a mentor.
534
00:34:02,860 --> 00:34:08,980
If you found the information in this episode to be helpful, don't keep it all to yourself.
535
00:34:08,980 --> 00:34:10,700
Someone else needs to hear it.
536
00:34:10,700 --> 00:34:14,760
So take a minute right now and share it.
537
00:34:14,760 --> 00:34:20,220
As you share this episode, you become part of our mission to help launch a new generation
538
00:34:20,220 --> 00:34:26,180
of clinician researchers who make transformative discoveries that change the way we do healthcare.
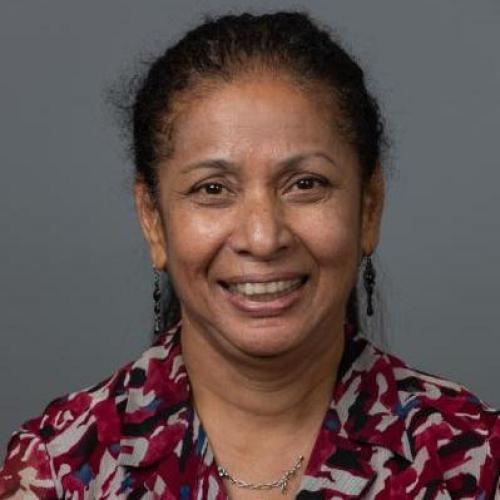
Maragatha Kuchibhatla
Professor
Maggie Kuchibhatla, PhD
Professor
Department of Biostatistics and Bioinformatics
Dept. of Psychiatry and Behavioral Sciences
Senior Fellow, Center for Aging and Human Development
Duke University Medical Center
Durham, NC